Clik here to view.
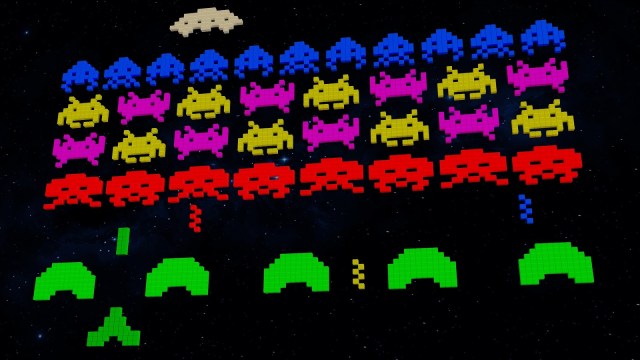
Clik here to view.
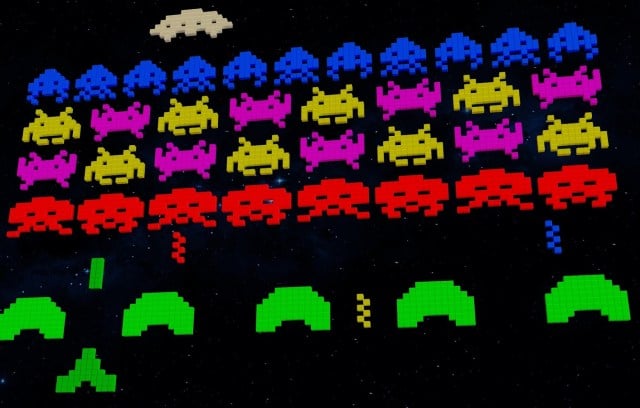
Introduction
- Environment: this describes the game in which the agent must act and learn to develop.
- Reward: the incentive that the agent obtains after carrying out a determined action. In the case of Breakout-v0, the agent receives a positive reward when it manages to return the ball and destroy one of the bricks.
- State: this is usually a tensor obtained from the observation space of the environment. In this case, the states consist in a collection of preprocessed images with the aim of helping to train the model.
- Action: this is a possible move in the action space that the agent can carry out, based on the current game state or the historic states that it has studied. For example, in our case it would be to move left, right or stay still in terms of direction, and to shoot the ball
- Control policy: this determines how the agent chooses the action that it will take. The programmer can choose the control policy at the time of carrying out the training of the neural network. Normally, you can choose a random action to start with, and once the model trains sufficiently, it will act based on the maximum value that the model has obtained up to that point.
Image may be NSFW. Clik here to view. ![]() |
Figure 1: Diagram showing the learning process of an agent during the training. |
Beginning the Training
Control Policy
When approximating the map of different states and actions, Reinforcement Learning techniques are often quite unstable when using a deep neural network. This is due to the nonlinearity of neural networks and the fact the small changes in Q-Values, when there is an inappropriate control policy, can drastically change the action and therefore lead to very different game states.
Due to all this, and with the aim of reducing instabilities that could arise during training, one usually runs a random sample of a large number of states, actions and rewards in order to explore the greatest number of possibilities of the current casuistry and avoid divergences and blockages in the model’s training.
.gist-file
.gist-data {max-height: 500px;}
Q-Function
The Loss and Optimization Functions
Given the large number of frames per second to process, and the elevated dimensionality of the game states, it is impractical to directly map the causality between action and state. This forces us to approximate the Q function through our random sample of states, rewards and actions.
Pre-Processing Input Data
One of the main deciding factors of a good training of the model, given the long computing times required, is the pre-processing of the image and the nature of the input to the neural network. This will also directly affect the routines that one needs to develop for interacting with the environment. In general, it is advisable to process the image generated by the Gym environment before it is included in the model. Generally, this aims to reduce its dimensionality, by eliminating the information that would not be useful when training the neural network. Normally, there would be an emphasis on the information relating to color that OpenAI Gym contains in its three color channels. These channels do not contain valuable information for the training of our model, and will therefore be forgotten before introducing the states to the model.
- As a first approximation, we take images of the game environment and process them; making them greyscale, resizing them, removing any background and using a simple image filtering to detect movement. The resulting state of these steps is the latest image of the environment as well as recent traces of movement of the objects.
- In the second alternative, we have opted for using a stack of four images as the input, with the intention of allowing the model to learn to detect movement. This is necessary since an individual state offers little information about the velocity and direction of the ball and paddle.
We are only interested in the area of the game where the ball and paddle are moving and where the bricks are. The borders of the screenshots do not offer valuable information to the model, so we eliminate these areas. Furthermore, we reduce the resolution of the image by 50% and turn it black and white (in a binary scale) since the RGB channels also offer little information of interest.
In the next post, we will offer a description of the architecture of the model with which he have trained our agents in Breakout-v0 and SpaceInvaders-v0. We will also explain in greater detail the logic of the training, explain the testing phase, and offer some conclusions about the project.
The post Deep Learning vs Atari: train your AI to dominate classic videogames (Part II) appeared first on Think Big.